In contemporary marketing, audience awareness is king. Consumers have been segmented by demographic, geography, and psychographics for decades—yet traditional methods are too often lacking in sophistication, agility, and promptness. That is no more.
Due to Artificial Intelligence, customer segmentation can now be done to a whole new extent. No more static segments or big audience segments for marketers to work with. AI systems can now analyze vast volumes of behaviorally driven data to discover hidden patterns and identify customer requirements and create super-accurate segments—both automatically and in real time.
In this article, we’re going to talk about how AI customer segmentation is revolutionizing target marketing by enabling companies to make more relevant, personalized and effective campaigns. We’re going to go behind the curtain to see how AI works and why it is better than traditional methods. We’ll also see how companies in different sectors are using it to drive ROI, loyalty, and expansion.
Understanding AI-Powered Customer Segmentation
Segmentation of a brand’s customer base into similar sets of characteristics is customer segmentation in a nutshell. Customer segmentation has been based on age, gender, geography, or buying behavior—manual-parameter-setting and blanketapply by campaign. While a great place to begin with personalization, this method was inevitably short-changed in reflecting variation and nuance of actual behavior.
What is AI-Powered Customer Segmentation?
AI segmentation uses machine learning and predictive analytics to filter through vast amounts of structured and unstructured information—such as buying behavior, web site behavior, social behavior on web sites and on social networks, mail behavior, and even support behavior. Unlike static segmentation models, AI can:
- Segment based on real behavior patterns in real-time.
- Continuously refine groups based on evolving customer behavior.
- Identify more subtle relationships that would elude conventional analysis.
How AI differs from traditional segmentation
Traditional Segmentation | AI-Powered Segmentation |
Based on fixed rules (e.g., age 18–25) | Based on dynamic behavioral data |
Updated manually | Continuously updated in real time |
Limited data types | Analyzes multi-source, multi-format data |
Generalized messaging | Hyper-personalized targeting |
Not only do AI examine what individuals undertake—but it also assists in looking forward to what they will do subsequently and how to position oneself for it.
It is important.
With consumers insisting on individual treatment, no longer do blanket campaigns do. AI-powered segmentation enables brands to:
Make sure to offer readers interesting content.
- Raise conversion rates. Reduce churn by forecasting discontent.
- Increase lifetime value based on improving customer journeys.
Key takeaway: AI-powered customer segmentation is not just more powerful—darker and smarter and more responsive—giving marketers a great advantage in a competitive and dynamic digital landscape.
The Benefits of AI-Powered Customer Segmentation
AI-powered customer segmentation is not only a clever way to divide your audience—It is revolutionary to utilize to build hyper-personal, data-backed experiences. From optimizing campaign efficacy to streamlining ROI, using AI to power segmentation has benefits that go far beyond basic personalization.
1. Increased Precision and Accurcy
AI can execute through enormous databases—such as real-time user behavior, purchasing behavior, and context clues—to identify patterns and connections that human analysts might not catch.
- Develops detailed customer segments based on actual behavior. It eliminates assumptions and subjectivity introduced by manual segmentation. Makes fact-based choices and not guesses.
For example, a retail brand using AI can know that consumers browsing a particular category late in the evening hours can be more prone to make a mobile buy—enabling you to promote to that audience with on-time mobile ads.
2. Real-Time Dynamic Segmentation
In comparison to typical methods, AI segmentation is dynamic—segments are refreshed as behavior evolves.
Tracked customer lifecycle activity in real time.
- Reallocates clients to the optimal category by default. It acts on recent trends, on-season activity, or external stimulus.
An airline can move a customer to a “high-end” segment from a “price-conscious” segment after two international bookings—triggering higher-tier promotions instantly.
3. Increased Personalization at Scale
Brands can use AI to go beyond basic demographics and target personalisation based on:
- Prior behavior and future behavior prediction.
- Television viewing patterns and program interests. The affect and emotional tone of the last messages. ???? One example: An ecommerce site can show different home page versions, emails, or products to thousands of micro-segments—without human intervention.
4. Maximising Campaign and Marketing ROI Effectiveness
AI assists in budgeting and resource allocation more effectively by:
- Addressing only those segments with customized messages.
- Reducing wastage on ineligible audiences.
- Increasing conversion rates through offering content precisely.
The company saw a 25% boost in lead conversion with AI-driven segmentation SaaS that was focused on behaviorally defined segments rather than broad interest-based audiences.
5. Increased Customer Satisfaction and CustomerRetention
By predicting imminent churn and pre-emptively segmenting vulnerable users, AI-powered segmentation assists businesses to:
- Engage inactive users before they are gone. Develop customized win-back programs.
- Reinforce loyalty through timely and pertinent touchpoints.
An example is a web site that can see reduced activity on a user watch history and recommend content based on past interests to get a user re-engaged.
Key takeaway: Customer segmentation through AI brings unparalleled precision in target accuracy, personalisation and responsiveness that allows marketers to move away from generic assumptions to deep and moment-by-moment customer insights that drive actionable results.
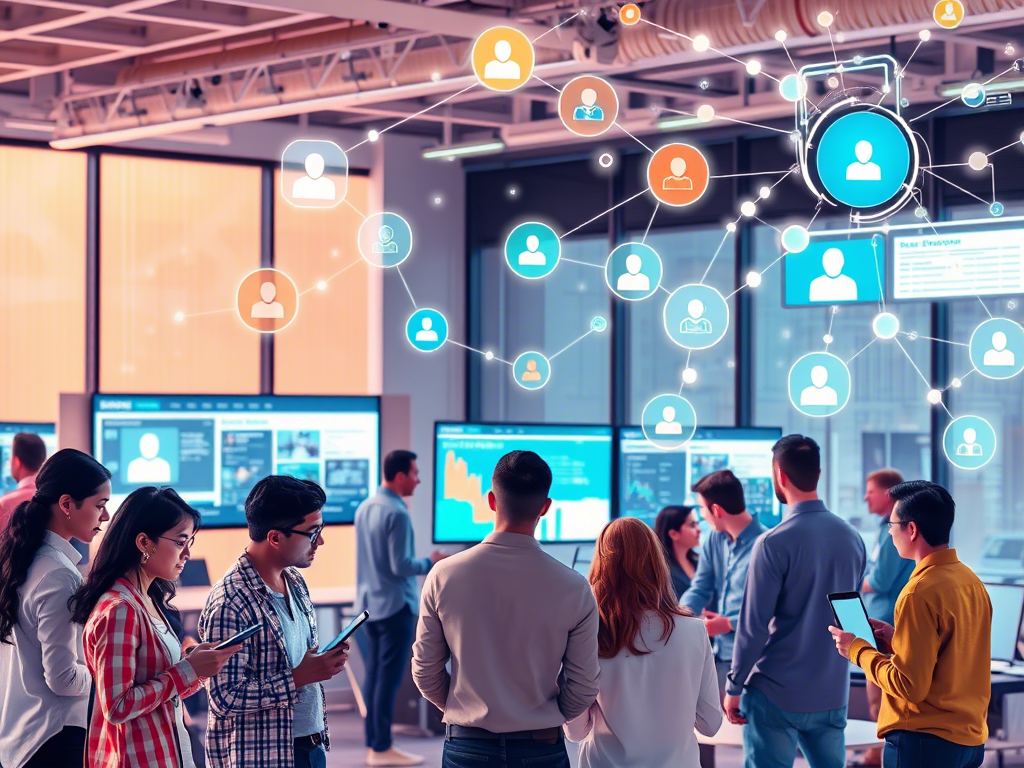
Real-World Examples of AI-Powered Customer Segmentation in Action
The potential of AI for customer segmentation is best exemplified by how top firms across each sector are using it to disrupt advertising. Actual case studies show that firms are using AI to achieve more targeted messaging, better conversions, and higher customer loyalty.
1. Shopping: AI-Powered Beauty by Sep
Sephora was required to discover how to make personalized recommendations to millions of consumers.
AI Platform: The company used an AI-powered engine that considered purchasing patterns, browsing patterns, test scores, and reviews for creating beauty profiles and segmenting consumers based on skin type, preferences, and what they need.
Outcomes Improved customer satisfaction through personalized products offered. Improved rates of conversion on desktop and mobile platforms. Improved loyalty through AI-customized skin care routines.
Key insight: AI-powered segmentation helped Sephora move away from mass marketing and toward individual-level personalizations.
2. Amazon E-commerce: Mastery of Micro
Since Amazon enjoyed a large and diversified audience size, it required it to target individual interests.
AI Solution: Amazon segments consumers based on behavior segmentation using machine learning by behavior patterns, purchasing intent, time to buy, and device usage and others. Amazon uses these micro-segments to serve personalized homepages, personalized emails, and recommendations on products.
Results: More engagement and retention with extreme-highly relevant targeting. Easy omnichannel personalisation across web, app, and mail. Increased average order value through smart bundling and cross-selling.
Takeaway: Amazon shows how segmentation by AI can drive personalisation across all customer experience.
3. Financial Services: American Express Predictive Segmentation
Task: American Express wanted to stay ahead of customer demand and reduce churn.
The company used AI-based predictive segmentation models to analyze spend behavior, lifestyle development, and financial patterns.
Output: Already identified key segments at high risk.
- Executed proactive retention campaigns with customized offers. Improved long-term customer value through offering personalized services.
Takeaway: Predictive segmentation enables data-driven decision-making with a direct impact on customer retention.
4. Streaming Services: Netflix’s Content Personalisation Engine
Having a large library to draw on, Netflix was challenged to match viewers with material they’d like.
Netflix uses AI to segment viewers by watch behavior, skip behavior, daypart and even by viewing based on emotion.
Discoveries Improved content discoverability through customized carousels. Increased viewing time and audience engagement.
- Reduced churn by ongoing match with evolving taste.
Takeaway: Netflix segmentation strategy shows that behavior can be used to create emotional connections based on content.
Key takeaway: Following are some examples of how AI-driven customer segmentation is not just theoretical—by and large, it is real and quantifiable and extremely scalable. From retail to finance to e-commerce to media, AI provides a way to greater relevance and greater ROI through smart segmentation.
Applying AI to your customer segmentation strategy
It doesn’t need to be daunting to get AI into your marketing stack. With the right strategy and tech, any size company can start using AI-powered customer segmentation to achieve more intelligent targeting, improved personalization, and more effective campaign efficiency. Here is how you can get it going:
1. Collect and Organize Quality Data
AI is only as good as you make it. Before you can utilize AI to your advantage, ensure that you:
Clean and consolidated customer information across all touch points. Not only demographics, but behavioral, transactional, and engagement data. Centralized Customer Data Platform (CDP)/ Customer Relationship Management (CRM) for processing inputs.
Pro tip: Leverage tools like Segment, Snowflake, or HubSpot to create your own efficient data gathering and amalgamation.
2. Choose Suitable AI Solutions and Tools
There are plenty of AI-powered segmentation options ranging from plug-and-play offerings to enterprise-grade platforms. Look for those that offer:
- Machine learning-based segmentation capabilities (including predictive rating and live clustering).
- Smooth coordination with your existing email, ad and analytics platforms.
- Transparent models that provide insight into why a customer was put into a segment.
Shared tools: Marketing Cloud of Salesforce, Real-Time CDP of Adobe, Optimove, Blueshift, and Segment Personas.
3. Align AI with Business Goals
Your segmentation strategy has to be connected to key objectives:
Improve lead quality. Optimize for behavior-based scores. Reduce churn. Develop predictive segments for those at risk.
- Increase LTV? Segment along axis of engagement or usage behavior.
For instance, a B2B SaaS company can segment by adoption of different products to identify possibilities for cross-selling.
4. Inject AI into Campaign Workflows
After segments are streamed, build them into your existing channels:
- Use dynamic content blocks to personalize email campaigns. Build customized web experiences based on behavior segmentation. Launch automated onboarding sequences for upsell or win-back campaigns.
Pro tip: Create feedback mechanisms in near real-time to enable AI models to learn and improve campaign performances.
5. Continuously monitor and optimize
AI models require ongoing modifications. Ensure that you:
Track KPIs including open rates, conversions and segmentation movements. Review segments regularly for functionality and applicability. Improve inputs and labeled data to refine AI forecasts with time.
If your segment is not optimal, retrain with greater behavior signals or remove noisy points.
Most important takeaway: AI incorporation into your customer segmentation strategy is not a project – it’s a constant evolution that expands with your goals, tools, and data. With adequate setup and mindset, incremental steps can achieve tangible gains in revenue, retention, and engagement.
AI-based marketing ethics and privacy concerns
While AI is used more and more for customer segmentation and target marketing, ethical accountability and data privacy should be placed on center stage. While all these technological advances create tremendous power to personalize, they also create significant questions regarding consumer trustworthiness, utilization of data and transparency.
1. Data Privacy and Compliance
AI segmentation relies on vast amounts of consumer information—buying behavior and browsing behavior—but with great power there is great responsibility.
- Ensuring total compliance with privacy legislations like GDPR and CCPA and other global standards. Make sure to reveal what you are collecting and for what reason. Make it possible for users to opt out or control individual preferences.
Best practice: Give straightforward and concise privacy statement and simple to use consent functionalities on all touch points.
2. Explainability and Transparency
It is difficult to say for sure why specific customers were segmented or targeted as AI is “black box” to them. Transparency is undermined by this obscurity.
- Leverage tools and platforms that offer transparent AI—those that tell you why a decision has been made.
- Steer clear of really complex or esoteric segmentation logic unless it’s testable and verifiable. Ensure marketing teams are aware of how AI-based segments are created and deployed.
Tip: Document and reveal AI segmentation criteria to internal stakeholders and privacy officers to ensure traceability.
3. Minimising Algorithmic Bias
AI is learned on accessible data that can be biased or based on incomplete sets. This can lead to discriminatory segmentation or biased aiming.
- Regularly audit your AI models for biased output—such as over-targeting by geography, gender, or income. Train models with big and representative sets to build equity. Involves human judgment in building or establishing segments.
For instance, a model that has a disproportionate emphasis on rich segments will by default exclude other segments that are worth serving.
4. Balancing Intrusion and Personalisation
While consumers value relevance, too personalized ads are pesky.
- Refrain from using too personal or specific points of information in messaging (such as “We saw that you viewed this product at 2 a.m.”).
- Prefer timely and relevant messaging to hyper-targeting that is too personalized. Make value-based personalisation—deliver useful content and avoid clickbait.
The general rule is that your personalization is creepy and worthless unless it is.
5. Ethical AI Governance
AI marketing campaigns should be included in a general ethical framework that includes:
- Definite internal policies for processing of data, consent and target. Cross-functional with legal and compliance and marketing teams.
- Ongoing training for marketing teams on ethical AI usage.
Tool tip: Leverage privacy-by-design platforms that restrict using sensitive data for segmentation by default.
Most important takeaway: AI segmentation can open up enormous marketing potential—but only with clear ethics, transparency, and respect for privacy. Marketers that get that right will see long-term trust and not just short-term clicks.
The Future of AI-Powered Customer Segmentation for Marketing
The greater AI grows and evolves, the greater will be the potential of customer segmentation. Using hyper-personalization or predictive modeling in real time is what that future holds for more perceptive and responsive and deeply intuitive segmentation systems based on AI.
1. Hypergranular behavior-based segments
AI will allow marketers to break through beyond simple segments and into micro-moments, with in-the-moment behavior dictating what is targeted.
- Segments by what is happening now or by immediate purpose.
- Relevant content presented in the right moment using the best possible medium. Make dynamic message and offer changes based on behavior by seconds.
An apparel retailer can offer a consumer browsing for sneakers on a phone during lunch with a instant deal for his/her size and style.
2. Anticipation and Forecasting Marketing
In place of responding to customer action, AI will enable companies to pre-empt desires before they are voiced.
- AI will predict what and when purchases are going to happen.
- Preemptive campaigns will be deployed by marketers with maximum possible conversion. Anticipatory targeting will be more a rule than an exception.
For instance, a holiday company can predict that a user is about to book holidays and serve location-based ads weeks ahead of booking time.
3. Integrations with Emerging Technologies
AI-powered segmentation shall become more interactive and productive with
- Voice and spoken word interfaces (such as Alexa, chatbots, smart TV). Virtual and augmented reality with personalized experience in man-made worlds. Wearables and IoT with segmentation based on physical activity, location and biometric.
The application can segment individuals on the quality of sleep by using a smartwatch and provide recommendations of content or products based on this.
4. Democratization of AI Tools
Now that AI is more accessible, even small businesses will:
- Leverage no-code or low-code AI platforms to create segmentation models.
- Rely on drag-and-drop interfaces to target with enterprise team accuracy. Compete on personalization, not on price or product.
Tip: Already existing tools like ChatGPT, Zoho or Jasper AI are bringing AI marketing to the doorstep of startups and SMEs.
5. Ethical AI as Competitive Advantage
In the future to come, privacy-first AI methods would be a brand differentiator.
Good segmentation will generate greater customer loyalty and trust.
- Respect for boundaries around information will make companies stand out in a competitive marketplace. AI that is ethical would be as crucial as efficiency in decision-making.
Prediction: Expect ethical AI certification or privacy badges to drive purchasing and user trust.
Bottom line: AI-powered future customer segmentation is about ethics, personalisation and prediction in real-time. Brands that leverage these developments won’t just become more effective at marketing—build long-term relationships with consumers.
Conclusion
AI-powered customer segmentation is not in the future anymore—today it is a marketing requirement. With predictive analytics and machine learning, companies can now see deeper into their audience through machine learning and predictive analytics and build super-personalized experiences and respond to consumer behavior in real time.
From improving campaign efficiency to inducing higher retention and reduced wastage spend, AI is transforming segmentation into dynamic behaviorally based insights instead of static demographics. With tools now accessible to them too, even smaller firms can benefit from it—not just the giants.
Key Points AI creates more granular, dynamic, and actionable customer segments. It enables real-time personalisation and predictive segmentation. Long-term success is going to be driven by AI ethics and open data practices.
- Companies that implement AI-powered segmentation now will be leaders in customer-centric marketing tomorrow.
Bottom line: To stay ahead in a expanding digital world, marketers need to leave behind traditional target practices. AI-powered segmentation is the future—the future is now.